Data-Based Decision Making in Assessment
Making informed decisions is paramount to enhancing student learning and academic success. Data-based decision making, a practice rooted in evidence and analysis, has emerged as a transformative approach to assessment.
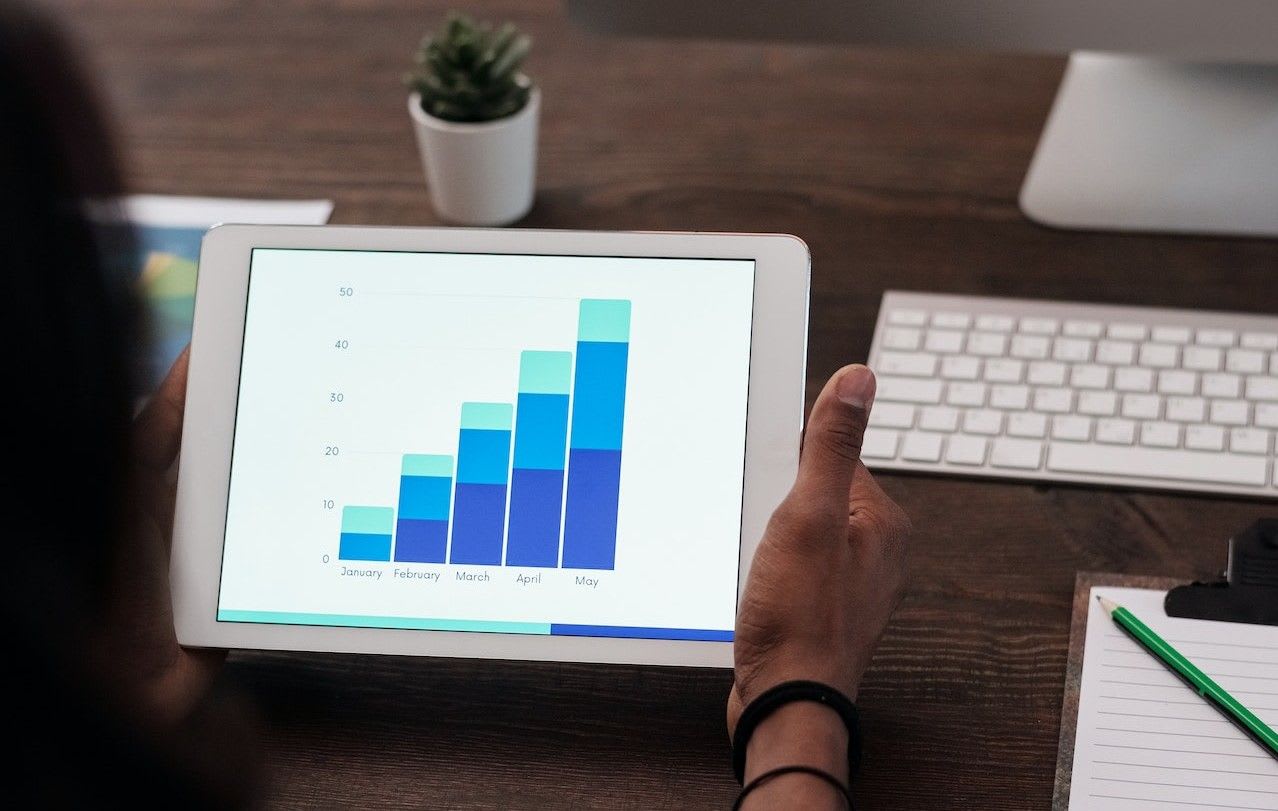
Making informed decisions is paramount to enhancing student learning and academic success. Data-based decision making, a practice rooted in evidence and analysis, has emerged as a transformative approach to assessment. This article delves into the realm of data-based decision making in assessment, exploring its significance, methodologies, and tangible impact on educational outcomes.
💡 Lessons learnt: Transforming education with insights, unlocks tomorrow's potential
Understanding Data-Based Decision Making
Data-based decision making involves the systematic collection, analysis, and interpretation of data to inform educational choices. It embraces the concept that assessment is not merely an end-point evaluation, but an ongoing process that provides valuable insights to educators, administrators, and policymakers. By harnessing the power of data, educators can tailor instruction to individual student needs, enhance curriculum design, and foster a culture of continuous improvement (Mandinach & Honey, 2008).
Effective data-based decision making begins with the collection of various types of data, including student performance on assessments, attendance records, and demographic information. This data is then analyzed to uncover patterns, trends, and areas of concern. Advanced data analytics tools allow educators to delve deeper, identifying correlations between different factors and understanding the root causes of student challenges (Datnow & Park, 2009).
Frameworks for Data-Based Decision Making in Assessment
Several frameworks for Data-Based Decision Making in Assessment have been developed to guide educators in effectively using data to inform instructional practices and enhance student outcomes. These frameworks provide structured approaches to collecting, analyzing, and utilizing data to make informed decisions. Here are a few prominent frameworks:
Data Wise Improvement Process: The Data Wise Improvement Process is a systematic approach developed by the Harvard Graduate School of Education that equips educators to effectively use data to drive instructional improvements and enhance student learning outcomes. The Data Wise Improvement Process places a strong emphasis on collaboration, ensuring that educators work together to analyze data, derive meaningful insights, and implement targeted strategies. Technological tools like data dashboards and visualization software enable educators to access real-time data, visualize trends, and collaboratively identify areas for improvement.
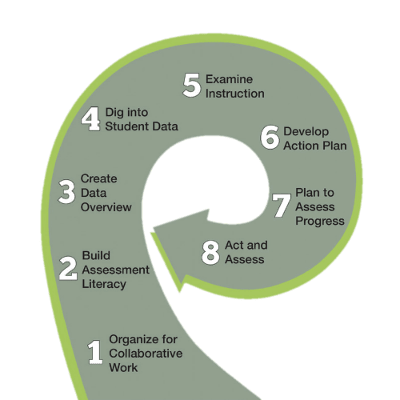
Assessment for Learning (AfL): AfL focuses on using assessment data to inform instruction in real-time. This student-centered framework shifts the focus from summative evaluation to a proactive approach, where assessments are viewed as tools. Teachers collect data through formative assessments, analyze it, and adjust teaching strategies accordingly. Technology tools like classroom response systems (clickers), digital quizzes, and class management systems facilitate quick data collection and immediate feedback, enhancing the efficacy of AfL.
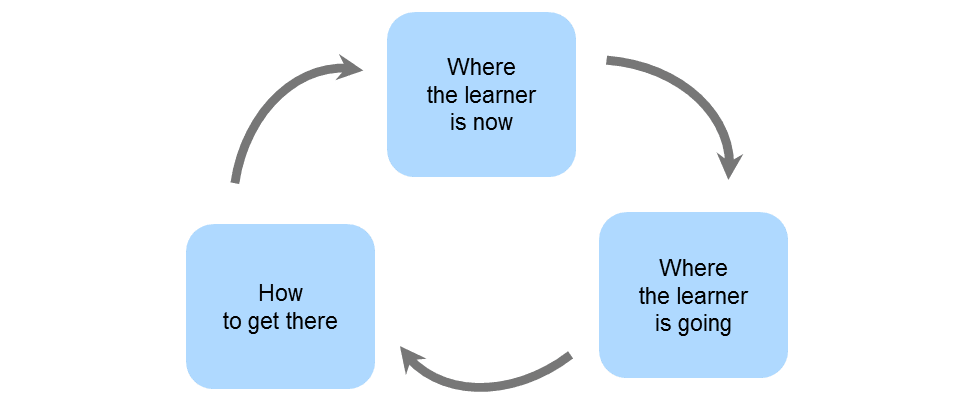
Response to Intervention (RTI): RTI is a multi-tiered framework designed to identify and support students with learning and behavior needs. RTI involves three tiers. It's main components include: Screening, school-wide screening or universal screening, Progress monitoring, Tiered instruction, High-quality, research-based instruction/interventions, Differentiated instruction and Fidelity of implementation. In this framework, educators identify students who are struggling academically or behaviorally and provide systematic interventions aligned with their specific challenges.
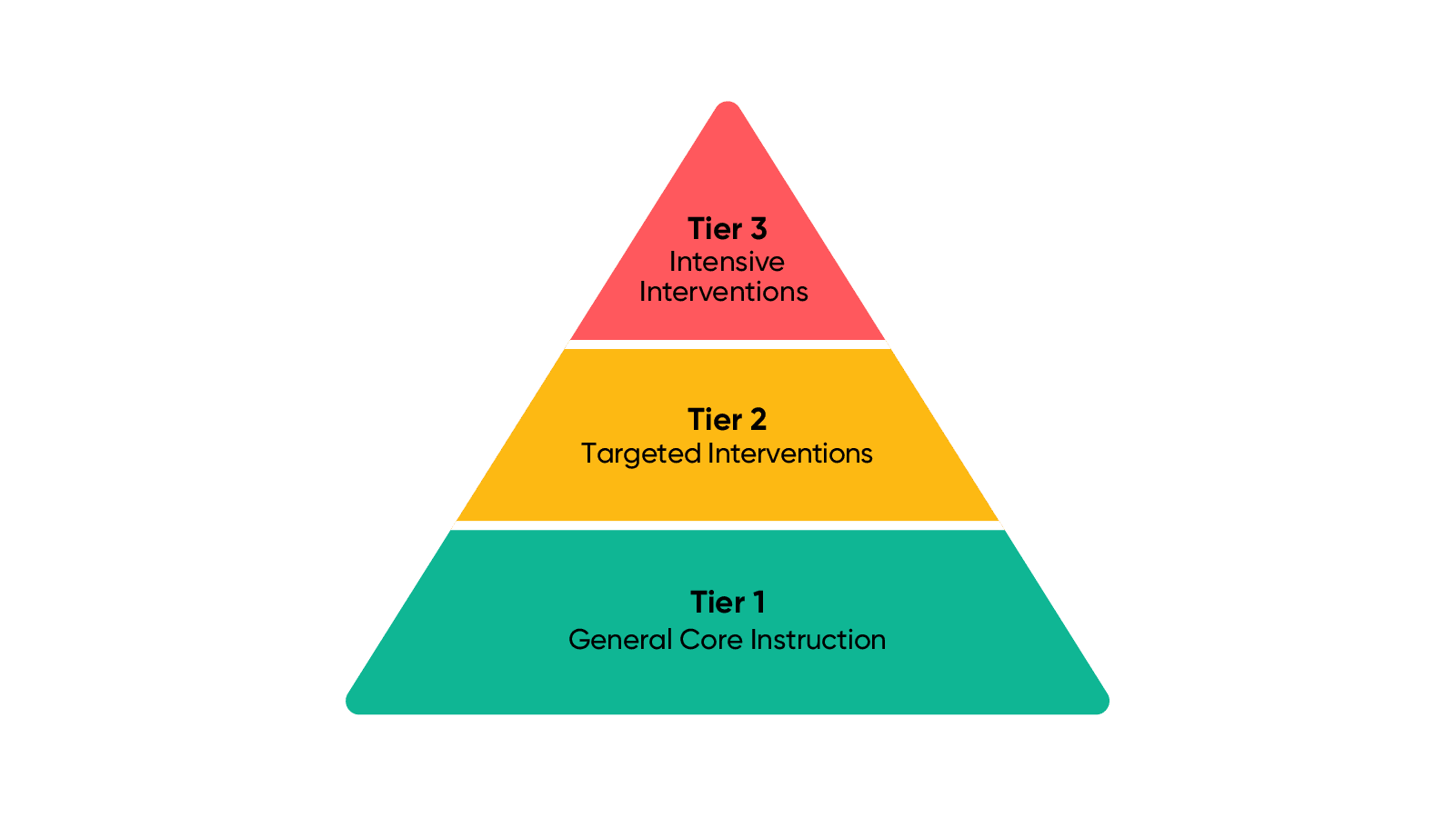
Targeted Interventions and Personalized Learning
Data-based decision making enables educators to implement targeted interventions that address specific student needs. For instance, if data reveals that a group of students is struggling with a particular math concept, educators can design interventions tailored to those challenges. This personalized approach fosters a supportive learning environment where students receive the assistance they require to succeed (Wayman et al., 2007).
Educators armed with data are empowered to make proactive decisions that impact both classroom instruction and school-wide policies. By identifying strengths and weaknesses in teaching strategies, educators can adjust their methods to better serve their students. Similarly, administrators can allocate resources effectively, track progress, and develop targeted professional development initiatives for their staff (Hamilton et al., 2009).
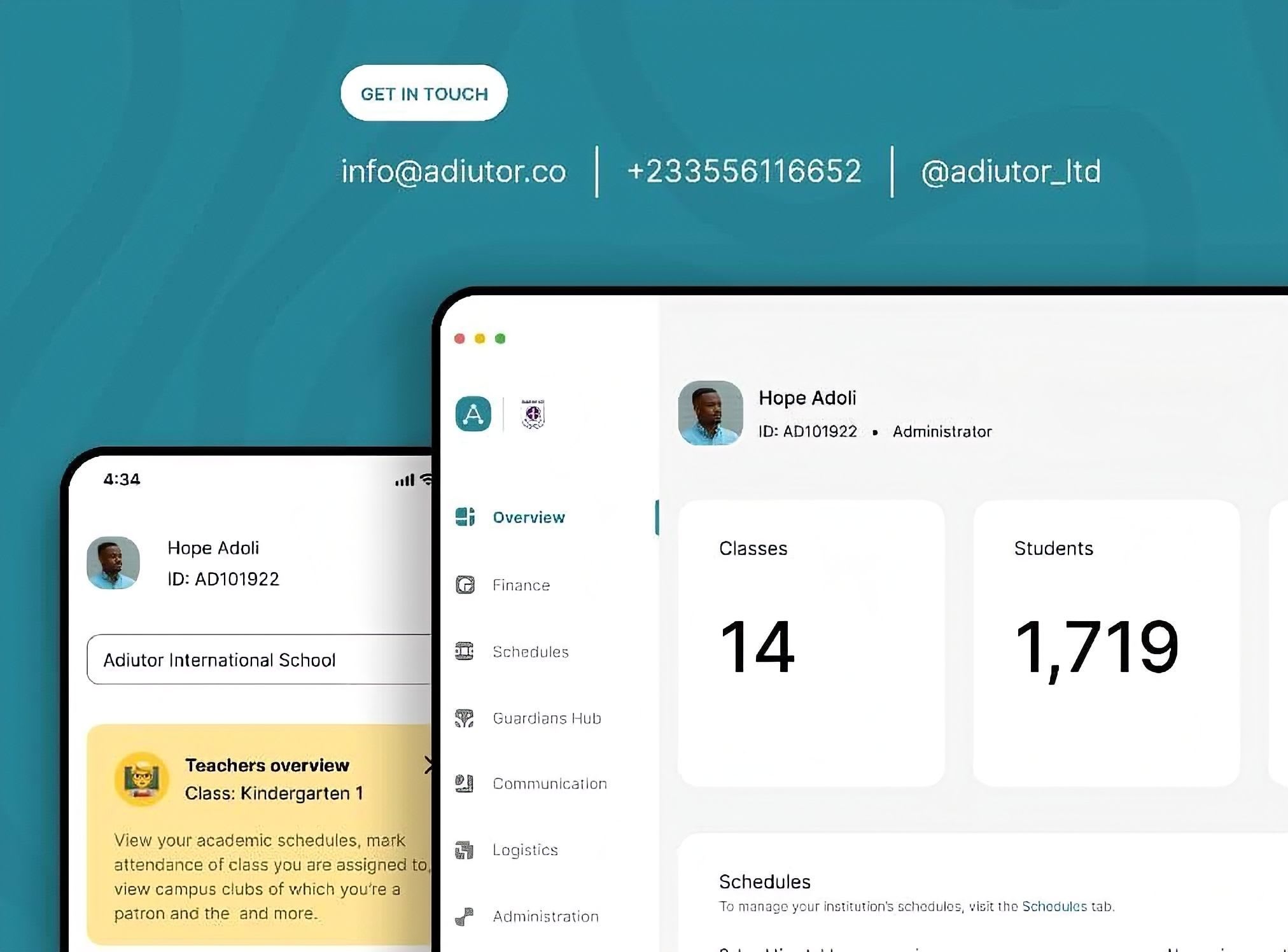
Adiutor
Adiutor means "helper" - we do just that, by taking a load of your school administration and helping you focus on what matters most: the kids.
References:
Allensworth, E. M., & Easton, J. Q. (2005). The On-Track Indicator as a Predictor of High School Graduation. Chicago, IL: Consortium on Chicago School Research.
Balfanz, R., Herzog, L., & Mac Iver, D. J. (2007). Preventing Student Disengagement and Keeping Students on the Graduation Path in Urban Middle-Grades Schools: Early Identification and Effective Interventions. Educational Psychologist, 42(4), 223-235. doi:10.1080/00461520701621025
Datnow, A., & Park, V. (2009). Data-driven Decision Making in the School District: Lessons from Urban School Leaders. Journal of School Leadership, 19(6), 632-664.
Hamilton, L. S., Halverson, R., Jackson, S. S., Mandinach, E., Supovitz, J. A., & Wayman, J. C. (2009). Using Student Achievement Data to Support Instructional Decision Making (NCEE 2009-4067). National Center for Education Evaluation and Regional Assistance (NCEE).
Mandinach, E. B., & Honey, M. (2008). Data-Driven School Improvement: Linking Data and Learning. Teachers College Press.
Wayman, J. C., Cho, V., Jimerson, J. B., & Spikes, D. D. (2007). Beyond the Indicators: An Integrated School-Level Model for Improving Student Engagement and Performance. Journal of Educational Research, 100(6), 366-381. doi:10.3200/JOER.100.6.366-382
Boudett, K. P., City, E. A., & Murnane, R. J. (2014). Data Wise, Revised and Expanded Edition: A Step-by-Step Guide to Using Assessment Results to Improve Teaching and Learning. Harvard Education Press.
Black, P., & Wiliam, D. (1998). Assessment and classroom learning. Assessment in Education: Principles, Policy & Practice, 5(1), 7-74.
Fuchs, D., & Fuchs, L. S. (2006). Introduction to Response to Intervention: What, Why, and How Valid Is It?. Reading Research Quarterly, 41(1), 93-99.
Mandinach, E. B., & Jackson, S. S. (2012). Developing a data infrastructure for a next-generation education system. Harvard Education Press.
Halverson, R., Luckin, R., Hosken, D., & Oliver, M. (2017). Conclusion: A Technology-Integrated Continuous Improvement Model. In Handbook of Design in Educational Technology (pp. 285-289). Routledge.